The Power of Open Source Image Annotation Tools
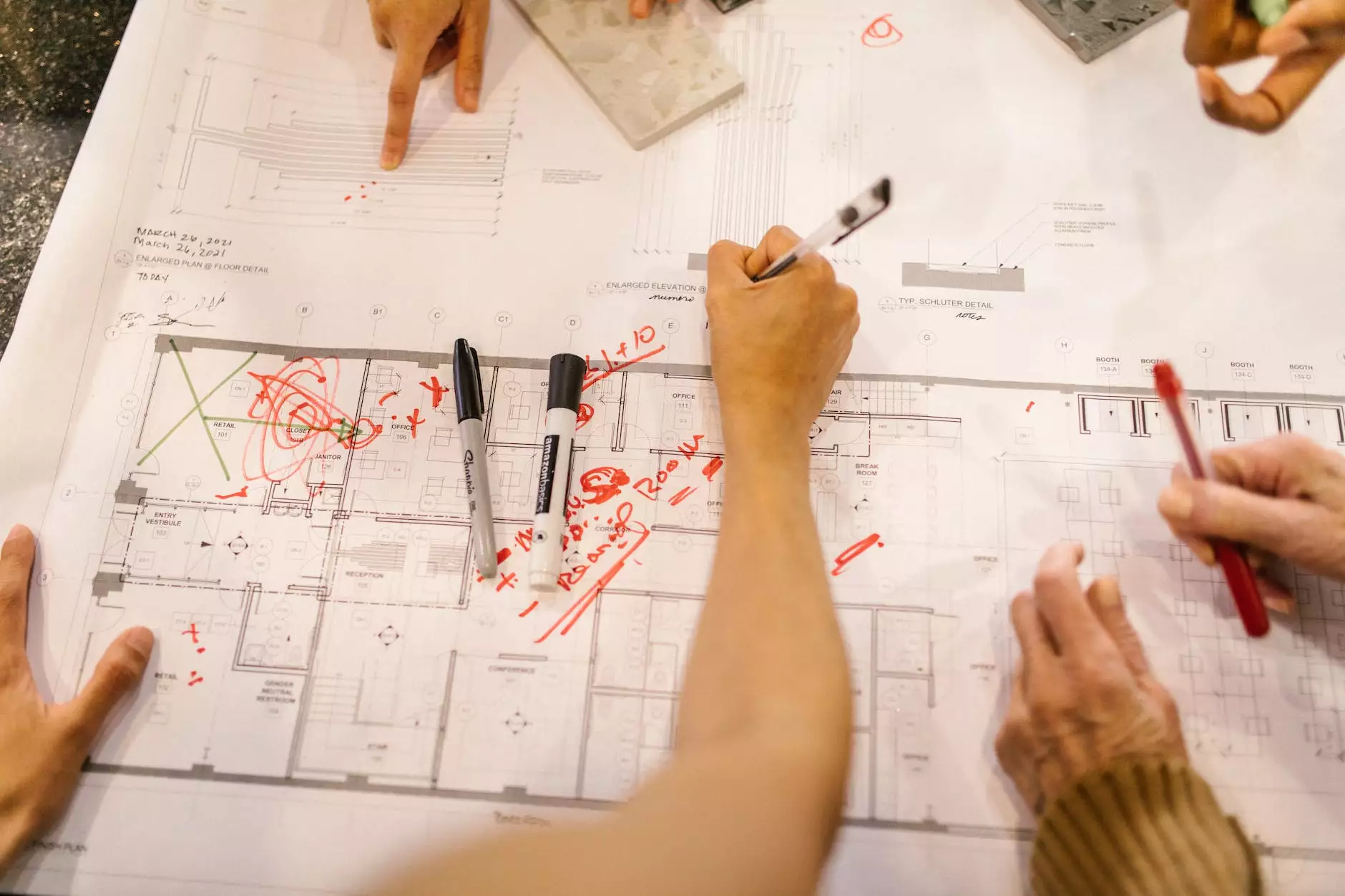
In the digital age, the significance of data is more pronounced than ever, and image annotation stands at the forefront of transforming raw visual data into actionable insights. Organizations, especially in domains like artificial intelligence, computer vision, and machine learning, are extensively leveraging data annotation tools to ensure that their AI models can accurately interpret images. Among these tools, open source image annotation tools are gaining traction, primarily due to their flexibility and cost-effectiveness.
What is Image Annotation?
Image annotation refers to the process of identifying and labeling objects within images, which is crucial for machine learning algorithms to understand visual content. This process not only aids in training AI models but also enhances their performance in real-world applications.
Benefits of Open Source Image Annotation Tools
Open source tools provide numerous advantages over proprietary software solutions. Below are some compelling reasons to consider open source annotation tools for your data annotation needs:
- Cost-Effectiveness: Open source tools are typically free to use, which is a significant advantage for startups and small businesses.
- Customization: Users have the ability to modify the tool's features to ensure it fits their specific needs. This level of flexibility is often absent in commercial products.
- Community Support: Open source projects usually have vibrant communities that provide support, updates, and improvements.
- Transparency: With open source software, users can inspect the code, ensuring data security and integrity.
- Collaboration: Many developers collaborate on open source projects, leading to rapid enhancements and updates.
The Growing Importance of Image Annotation in AI Development
With the rise of AI applications, the demand for accurate and efficient data annotation is skyrocketing. Image annotation plays a pivotal role in various AI-driven projects:
- Autonomous Vehicles: Precise labeling of pedestrians, traffic signs, and other vehicles is essential for the training of self-driving technologies.
- Healthcare: Annotated images are critical for diagnostic AI systems, enabling them to recognize patterns in clinical imagery.
- Retail: Image recognition tools provide insights into consumer behavior by analyzing shop images and customer interactions.
- Security: Surveillance systems use annotated images to detect and identify suspicious activities consistently.
Top Open Source Image Annotation Tools to Consider
There is a myriad of open source image annotation tools available today. Here are some of the top contenders that can help streamline your annotation processes:
1. LabelImg
LabelImg is a popular graphical image annotation tool written in Python and uses Qt for its graphical interface. It's designed for easy image labeling and supports both Pascal VOC and YOLO formats. Its features include:
- Simple and customizable interface
- Supports multiple image formats
- Ability to create bounding boxes around selected objects
2. CVAT (Computer Vision Annotation Tool)
CVAT is developed by Intel and is tailored for professional use and complex annotation tasks. It allows users to annotate images and videos effectively with the following features:
- Support for multiple annotation types including bounding boxes, polygonal segmentation, and keypoint annotations.
- User-friendly web interface with robust collaborative features.
- Integration with machine learning workflows.
3. VGG Image Annotator (VIA)
The VGG Image Annotator is a simple and effective tool for annotating images and videos. Its unique features include:
- Lightweight and can be run locally in a user’s web browser.
- Supports various annotation types such as bounding boxes, circles, and polygons.
- Ability to save annotations in multiple formats.
Key Features to Look For in Open Source Annotation Tools
When selecting an image annotation tool, consider the following features to ensure it meets your organization's needs:
- Ease of Use: An intuitive interface is essential for efficiency, especially when working with large datasets.
- Annotation Flexibility: Support for different types of annotations like bounding boxes, segmentation, and keypoints enhances functionality.
- Collaboration Tools: Features that facilitate team collaboration and workflow are crucial for larger teams.
- Integration Capabilities: Look for tools that can seamlessly integrate with your existing data pipelines and machine learning frameworks.
- Export Options: Multiple export formats ensure that your annotations can suit various machine learning models.
Best Practices in Image Annotation
To achieve optimal results in your data annotation efforts, it's essential to follow best practices. Here are some guidelines:
- Define Clear Annotation Guidelines: Provide annotators with clear instructions and examples to ensure consistency across the board.
- Quality Control: Implement a review process to verify annotations, as mistakes can significantly hinder machine learning model accuracy.
- Utilize Pre-trained Models: Consider leveraging pre-trained models for semi-supervised annotation to speed up the annotation process.
- Iterate on Feedback: Regularly collect feedback from your annotators and make adjustments to your guidelines and tools as necessary.
Conclusion: The Future of Image Annotation Tools
The landscape of technology is ever-evolving, and the demand for efficient data annotation solutions continues to rise. The growing community around open source image annotation tools is pushing the boundaries of what's possible in data preparation for AI.
These tools not only democratize access to powerful image annotation capabilities but also foster collaboration among developers and organizations. As the field of machine learning progresses, it's vital for businesses, especially those like Keylabs.ai, that specialize in data annotation tools and data annotation platforms, to embrace these tools to stay competitive.
By prioritizing effective data annotation strategies and leveraging the power of open source solutions, companies can enhance their machine learning efforts, resulting in more accurate and efficient AI applications that will revolutionize industries across the board.
In conclusion, image annotation tool open source options are not just alternatives—they represent a significant leap toward greater flexibility, community collaboration, and innovation in the realm of data annotation.